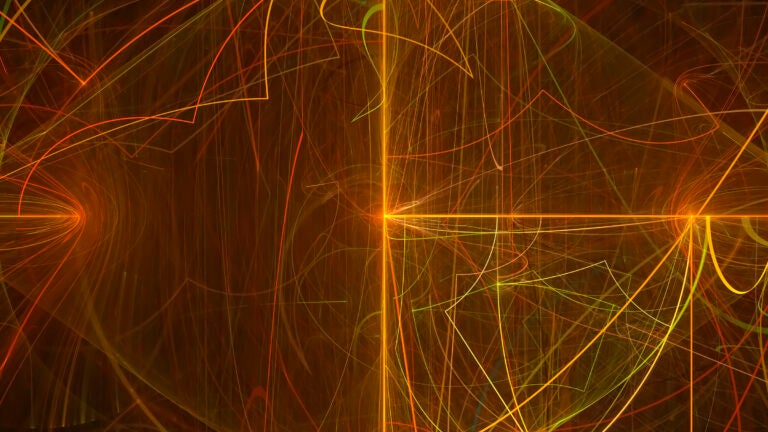
Image credit: iStock
Quantum computer outperforms supercomputers in approximate optimization tasks
USC-led study demonstrates the first quantum scaling advantage for approximate optimization problem-solving using a quantum annealer.
Contact: Will Kwong, willkwon@usc.edu; USC Media Relations, uscnews@usc.edu or (213) 740-2215
A quantum computer can solve optimization problems faster than classical supercomputers, a process known as “quantum advantage” and demonstrated by a USC researcher in a paper recently published in Physical Review Letters.
The study shows how quantum annealing, a specialized form of quantum computing, outperforms the best current classical algorithms when searching for near-optimal solutions in complex problems.
“The way quantum annealing works is by finding low-energy states in quantum systems, which correspond to optimal or near-optimal solutions to the problems being solved,” said Daniel Lidar, corresponding author of the study and professor of electrical and computer engineering, chemistry, and physics and astronomy at the USC Viterbi School of Engineering and the USC Dornsife College of Letters, Arts and Sciences.
Approximate optimization
Scientists have been struggling to demonstrate quantum scaling advantage (where the quantum advantage grows as the problem size increases) by the use of a quantum annealer for years. Quantum annealing has long been theorized to offer computational advantages for optimization, but definitive evidence of scaling improvements over classical methods has been elusive. This study shifts the focus from exact optimization (where quantum advantage remains unproven) to approximate optimization, an area with broad applicability in industry and science.
Quantum annealing is a specific type of quantum computing that can use quantum physics principles to find high-quality solutions to difficult optimization problems. Rather than requiring exact optimal solutions, the study focused on finding solutions within a certain percentage (≥1%) of the optimal value.
Many real-world problems don’t require exact solutions, making this approach practically relevant. For example, in determining which stocks to put into a mutual fund, it is often good enough to just beat a leading market index rather than beating every other stock portfolio.
To demonstrate algorithmic quantum scaling advantage, the researchers used a D-Wave Advantage quantum annealing processor, a specialized type of quantum computing device installed at USC’s Information Sciences Institute. Like with all current quantum computers, noise plays a major role in spoiling quantum advantage in quantum annealing.
To overcome this problem, the team implemented a technique called quantum annealing correction (QAC) on the D-Wave’s processor, creating over 1,300 error-suppressed logical qubits. This error suppression was key to achieving the advantage over parallel tempering with isoenergetic cluster moves (PT-ICM), the most efficient current classical algorithm for comparable problems.
‘Time-to-epsilon’ performance
The study demonstrated quantum advantage by utilizing several research methods and focused on a family of two-dimensional spin-glass problems with high-precision interactions. “Spin-glass problems are a class of complex optimization challenges that originate from statistical physics models of disordered magnetic systems,” Lidar said. Instead of seeking exact solutions, the researchers benchmarked “time-to-epsilon” performance, measuring how quickly each approach could find solutions within a specified percentage of the optimal answer.
The researchers aim to extend their findings to denser, higher-dimensional problems and explore applications in real-world optimization. Lidar said further improvements in quantum hardware and error suppression could amplify the observed advantage. “This opens new avenues for quantum algorithms in optimization tasks where near-optimal solutions are sufficient.”
# # #
About the study: The study was co-authored by Humberto Munoz-Bauza of the NASA Ames Research Center and Lidar.
The study was supported by: Defense Advanced Research Projects Agency (DARPA) Grants HR00112190071 and NASA-DARPA SAA2-403688, U.S. Army Research Office Grant W911NF2310255, NASA.